
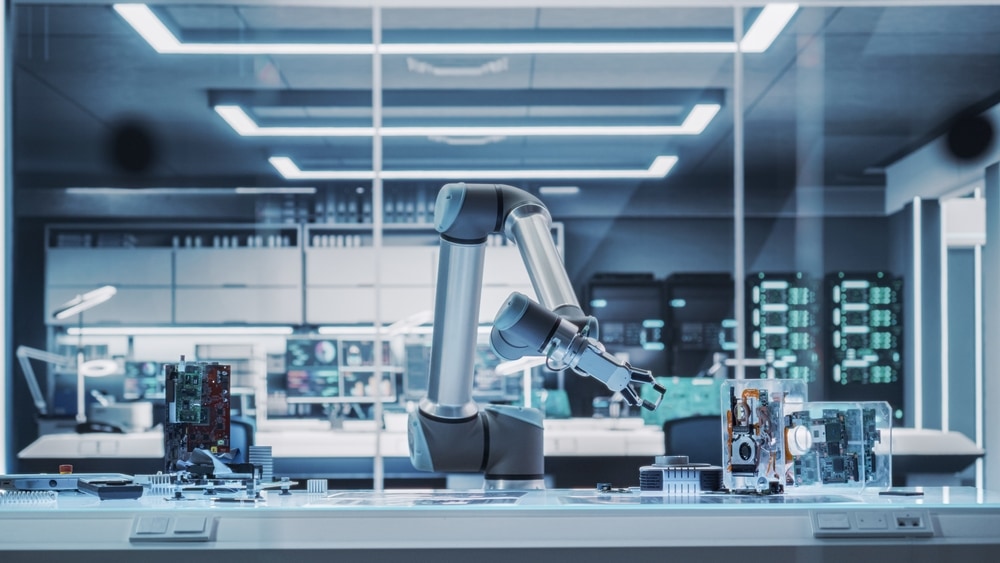
In the ever-evolving landscape of pharmaceuticals, the process of drug discovery remains a formidable challenge. However, with the advent of next-generation deep learning models, there is newfound hope for revolutionizing this crucial aspect of healthcare. These advanced AI-driven techniques are paving the way for faster, more efficient, and targeted drug discovery processes, offering unprecedented opportunities for innovation and breakthroughs in medical science. In this blog, we’ll explore the transformative potential of next-gen deep learning models in enhancing drug discovery in pharmaceuticals, and how they are reshaping the future of medicine.
Unraveling the Complexity of Drug Discovery:
Drug discovery is a complex and time-consuming endeavor that involves identifying potential compounds or molecules with therapeutic properties, testing their efficacy and safety, and ultimately bringing them to market as viable treatments. Traditionally, this process has relied heavily on empirical testing and trial and error, resulting in significant time and resource investment. However, with the emergence of next-gen deep learning models, there is a paradigm shift underway. These advanced Generative AI in Healthcare algorithms can analyze vast amounts of biological and chemical data, identify potential drug candidates, and predict their properties and interactions with unprecedented accuracy and efficiency. By leveraging the power of deep learning, pharmaceutical researchers can expedite the drug discovery process, accelerating the pace of innovation and bringing new treatments to patients more rapidly than ever before.
Enhancing Target Identification and Validation:
One of the key challenges in drug discovery is identifying and validating suitable targets for therapeutic intervention. Next-gen deep learning models are revolutionizing this aspect of the process by enabling researchers to analyze complex biological datasets and identify potential drug targets with greater precision and accuracy. By leveraging advanced machine learning techniques, such as neural networks and deep neural architectures, these models can sift through vast amounts of genomic, proteomic, and clinical data to identify key biological pathways and molecular targets associated with disease. This allows pharmaceutical researchers to focus their efforts on developing drugs that target the underlying mechanisms of disease, ultimately leading to more effective and targeted treatments.
Predicting Drug-Target Interactions with Precision:
Another critical aspect of drug discovery is predicting how potential drug candidates will interact with their intended targets in the body. Next-gen deep learning models excel in this area by leveraging advanced algorithms to analyze the structural and functional properties of both drugs and biological targets, predicting their interactions with remarkable accuracy. By training these models on large databases of known drug-target interactions, researchers can develop predictive models that can anticipate how new drug candidates will behave in vivo, helping to prioritize promising compounds for further testing and development. This predictive capability not only streamlines the drug discovery process but also reduces the likelihood of costly and time-consuming clinical failures, ultimately leading to more successful drug development pipelines.
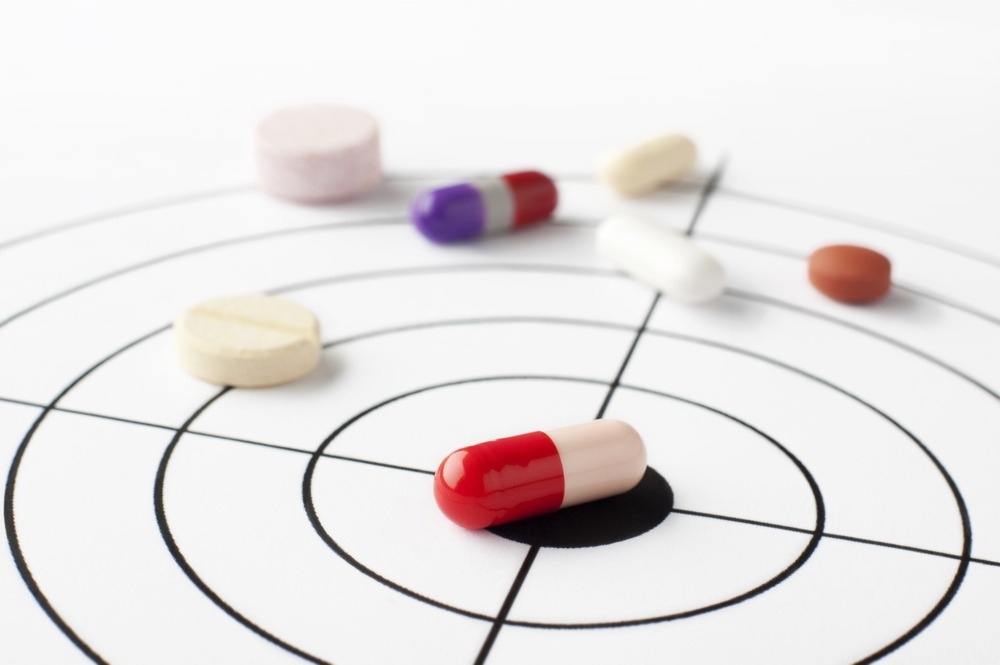
Advancing Drug Repurposing with Deep Learning:
One promising application of next-gen deep learning models in drug discovery is the repurposing of existing drugs for new therapeutic purposes. Traditional drug discovery approaches often involve the development of entirely new compounds, which can be time-consuming and costly. However, deep learning models can analyze large datasets of drug-target interactions and biological pathways to identify existing drugs that may have potential therapeutic effects for other diseases. By repurposing existing drugs, researchers can bypass many of the challenges associated with developing new compounds, potentially accelerating the process of bringing treatments to market. This approach not only saves time and resources but also offers new hope for patients with unmet medical needs.
Addressing Drug Resistance and Treatment Failures:
Drug resistance is a significant challenge in the field of medicine, particularly in the context of infectious diseases and cancer. However, next-gen deep learning models offer new strategies for addressing this challenge by predicting and understanding mechanisms of drug resistance. By analyzing genomic and proteomic data from resistant strains or tumor cells, these models can identify key molecular pathways and biomarkers associated with resistance, allowing researchers to develop more effective treatments that can bypass or overcome resistance mechanisms. Additionally, deep learning models can predict patient response to treatment, enabling personalized learning medicine approaches that tailor treatments to individual patients based on their unique genetic makeup and disease characteristics.
Unlocking the Potential of Drug Combination Therapy:
Combination therapy, which involves using multiple drugs to target different aspects of a disease simultaneously, has emerged as a promising strategy for improving treatment outcomes and overcoming drug resistance. Next-gen deep learning models are playing a crucial role in unlocking the potential of combination therapy by identifying synergistic drug combinations that can enhance therapeutic efficacy while minimizing adverse effects. By analyzing large datasets of drug interactions and molecular pathways, these models can predict which combinations are most likely to be effective against specific diseases, guiding researchers in the development of novel combination therapies. This approach holds immense potential for improving patient outcomes and transforming the way we treat complex diseases such as cancer, infectious diseases, and neurological disorders.
Collaborative Drug Discovery and Open Science Initiatives:
In the spirit of open science and collaboration, many researchers and organizations are leveraging next-gen deep learning models to advance drug discovery through collaborative efforts and open-access initiatives. By sharing data, tools, and insights, researchers can accelerate the pace of discovery and overcome barriers to innovation. Additionally, deep learning models can facilitate the integration of diverse datasets from different research groups and institutions, enabling more comprehensive analyses and enhancing the reproducibility of research findings. Through collaborative drug discovery initiatives, researchers can pool their resources and expertise to tackle some of the most pressing challenges in medicine, ultimately leading to more impactful discoveries and improved patient care.
Conclusion:
In conclusion, the integration of AI chatbot development services into the field of drug discovery represents a significant step forward in pharmaceutical research. These services offer invaluable support in streamlining communication, enhancing collaboration, and providing real-time assistance to researchers and clinicians. By leveraging AI chatbots, pharmaceutical companies can improve efficiency, reduce costs, and accelerate the pace of drug discovery efforts. Moreover, AI chatbots can facilitate the exchange of knowledge and insights among researchers, fostering a collaborative environment that fuels innovation and breakthroughs in medical science. As the pharmaceutical industry continues to embrace AI chatbot development services, we can anticipate further advancements in drug discovery and the development of life-saving treatments for patients worldwide.